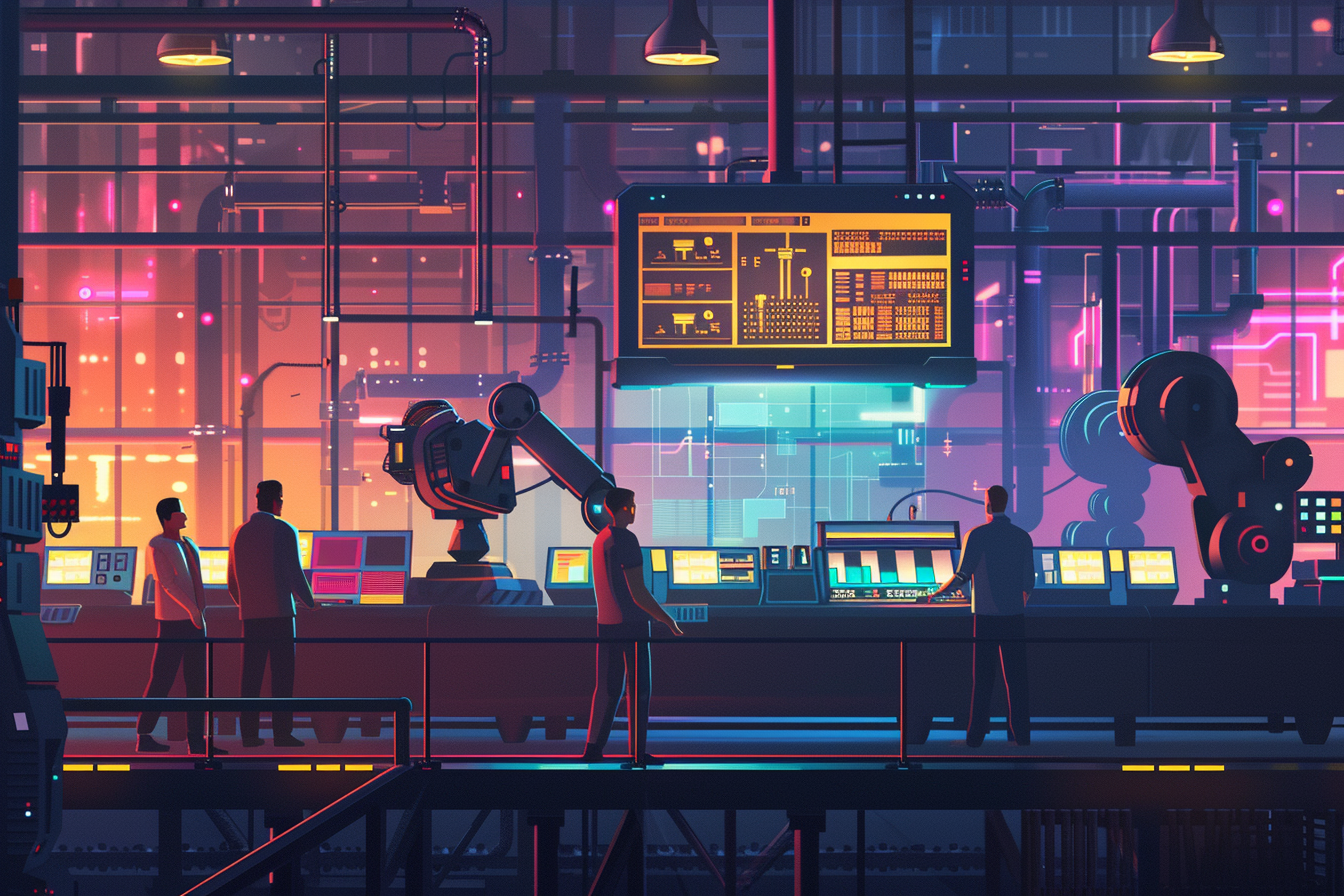
I recently examined where generative AI provides new early-stage investment opportunities. This month, we will explore opportunities in vertical applications, including some of the AI tooling that enables these opportunities.
The opportunity in Industry 4.0
The notion of a “Fourth Industrial Revolution” is not new. But it has had a fresh jolt of energy with the ascendance of the new LLMs. In essence, Industry 4.0 is the vision that we can use IoT-enabled sensors together with AI and ancillary technologies to bring the next level of automation to the way we manufacture things.
When people talk about Industry 4.0, it’s often defined narrowly, i.e., the manufacturing of goods. We think the idea of Industry 4.0 applies almost everywhere we make things – e.g. agriculture, biotechnology, energy (to name a few), but leave those aside for now. Let’s focus more specifically on manufacturing.
At the heart of the fourth industrial revolution lies the idea of autonomous systems. I.e. systems that can govern and regulate themselves, leading to a highly flexible and efficient production environment.
To understand how this improves manufacturing, let’s look at how things are done today.
How are machines operated today?
Modern manufacturing automates large parts of the production process. However, it is still reliant on a surprising amount of manual input from machine operators and other manufacturing staff. This means that expert operators configure things like:
- speed settings for conveyors, motors, or pumps,
- pressure settings in hydraulic or pneumatic systems,
- feed rates for material inputs,
- temperature setpoints etc.
Even when these have been configured to perfection by expert technicians, machines need to be recalibrated so machines keep working optimally. Reasons include:
- Wear and Tear:
- Mechanical Components: Constant movement and friction cause wear on gears, bearings, slides, and other mechanical parts. This wear subtly changes dimensions and tolerances, leading to inaccuracies in the machine’s output.
- Sensors and Tooling: Sensors degrade over time and tooling (cutting tools, molds, etc.) dull with use. This affects the machine’s ability to measure or process materials accurately.
- Environmental Factors:
- Temperature Fluctuations: Changes in temperature cause the expansion and contraction of machine components and materials being processed, which can lead to dimensional inaccuracies.
- Humidity: Affects certain materials and sensors, potentially impacting tolerances.
- Vibrations: Floor vibrations or internal vibrations from the machine’s operations can misalign components and affect its precision.
- Process Variability:
- Material Variations: Slight changes in raw material properties (hardness, density, etc.) can impact the forces and behaviours during machining, leading to a need for adjustment.
- Batch-to-batch Differences: Even when using the same materials, variations between batches can necessitate fine-tuning to maintain consistent quality.
- Quality Control & Maintenance:
- Regular Calibration Schedules: To ensure product quality, machines are usually placed on routine calibration checks to catch any drift out of tolerance.
- Post-Maintenance Recalibration: Any time a machine undergoes maintenance or replacement of parts, recalibration is crucial to re-establish its baseline accuracy.
To all this comes the effort that goes into manual inspection of finished products, the physical logistics of warehousing and distributing goods and the manual work that goes into overseeing the production process.
Why hasn’t manufacturing already been made fully autonomous?
Everyone who’s been close to manufacturing can see that there are innumerable opportunities to optimise and make better.
So why haven’t we already put an “AI megabrain” in charge of running all our plants? Some of the reasons include:
- Heterogenous machine parks. Many factories have machines that have been acquired in various installations over decades. Different models, different makes, differering levels of connectivity. Replacing everything with new “top of the line”, connected equipment requires an inordinate amount of capex. Even if the business case for automation on a greenfield site is good, it is often too costly to implement on an existing machine park.
- Subtleties and complexities of autonomous operations. Modern manufacturing has many, many variables (as outlined above). In many situations, there is no simple algorithmic way to optimise machine operations. Getting to proper autonomous operations requires quite sophisticated AIs.
- Data privacy. Many manufacturers have been reluctant to let manufacturing data leave their plants. The data may contain trade secrets that can be valuable in the hands of competitors. This has, in turn, meant that machine manufacturers have lacked the data they need to train AI models, making it harder to develop the next level of automation.
So, there is a big opportunity in manufacturing, but until now, there has been no easy way to unlock this. This is all changing with a confluence of new technologies coming to market.
How does next-generation AI enable autonomous manufacturing?
Here are four specific technology advances in and around AI that enable higher productivity in manufacturing:
- Federated learning solves the problem of how to train with proprietary data. Manufacturers are often hesitant to let manufacturing data leave their factories. But each factory doesn’t in itself create enough data to train robust models – you need data from many factories to build autonomous systems. Federated learning is a relatively new development in AI tha makes it possible to train and enhance AI models locally at the factory, to only send model improvements back to the “mothership”. In this way, the proprietary data can stay private, the AI models can benefit from the input of many factories at the same time.
- More robust AI models make it easier to retrofit existing plants. The more homogenous a factory, the easier it is to automate. But as we discussed above, the world is far messier. Once plants start operating, homogeneity quickly gives way for the messy reality of a shop floor. And that is not even taking into account all the factories that have equipment brought together from many vendors. The smarter AI models we have, the easier it is to provide “all purpose” automation upgrades, because the AI will be resilient enough to take all the small and big variations into account. Federated learning helps, as do general advances in AI (including generative AI).
- Cheaper access to data. The past 15 years have seen massive decreases in the cost of sensors and cameras – in particular driven by the smart-phone revolution. Ubiquitous and inexpensive sensors and cameras make it possible to see and measure everything so we get the data we need. This was a big enabler as it helps provide access to training data. The approaches we mentioned above now helps unlock the value of that data.
- A much faster journey from idea to production. Generative foundation models make it much easier to go from concept to production model. And technologies like additive manufacturing (3D printing) help us more quickly test prototypes and even go to production ready models.
These are not alone. There are so many incredible developments right now that promise to give us a better and smarter way to make things. We are excited to back amazing founders from companies like Octaipipe, Ai Build, Hirundo and ThingTrax who work hard to bring the vision to reality, and we always look to meet more founders who are determined to transform how the world works and how things are made.